What is Decision Intelligence?
This guide introduces decision intelligence — what it does, why it’s different, and where it delivers value across the enterprise.
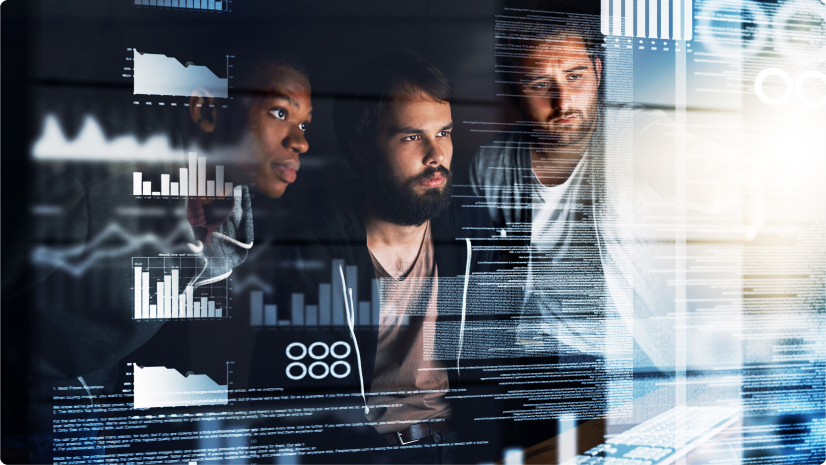
What is decision intelligence?
Decision intelligence (DI) is the optimization and orchestration of decision-making across an enterprise value chain. DI understands how decisions are made and uses data, analytics, AI, and automation to create a feedback-driven process that refines decisions over time — enhancing both decision quality and business impact through recommendations or autonomous action. In effect, DI shifts decision-making from people making decisions supported by machines to machines making decisions guided by people, allowing human oversight while dramatically increasing scale and speed.
Industry analyst IDC defines decision intelligence as being “a discipline and technology that helps organizations design, engineer, and orchestrate decisions by fully or partially automating all the steps in the decision-making process.” Similarly, Gartner has defined it as “the practical discipline used to improve decision making by explicitly understanding and engineering how decisions are made, and how outcomes are evaluated, managed and improved by feedback.”
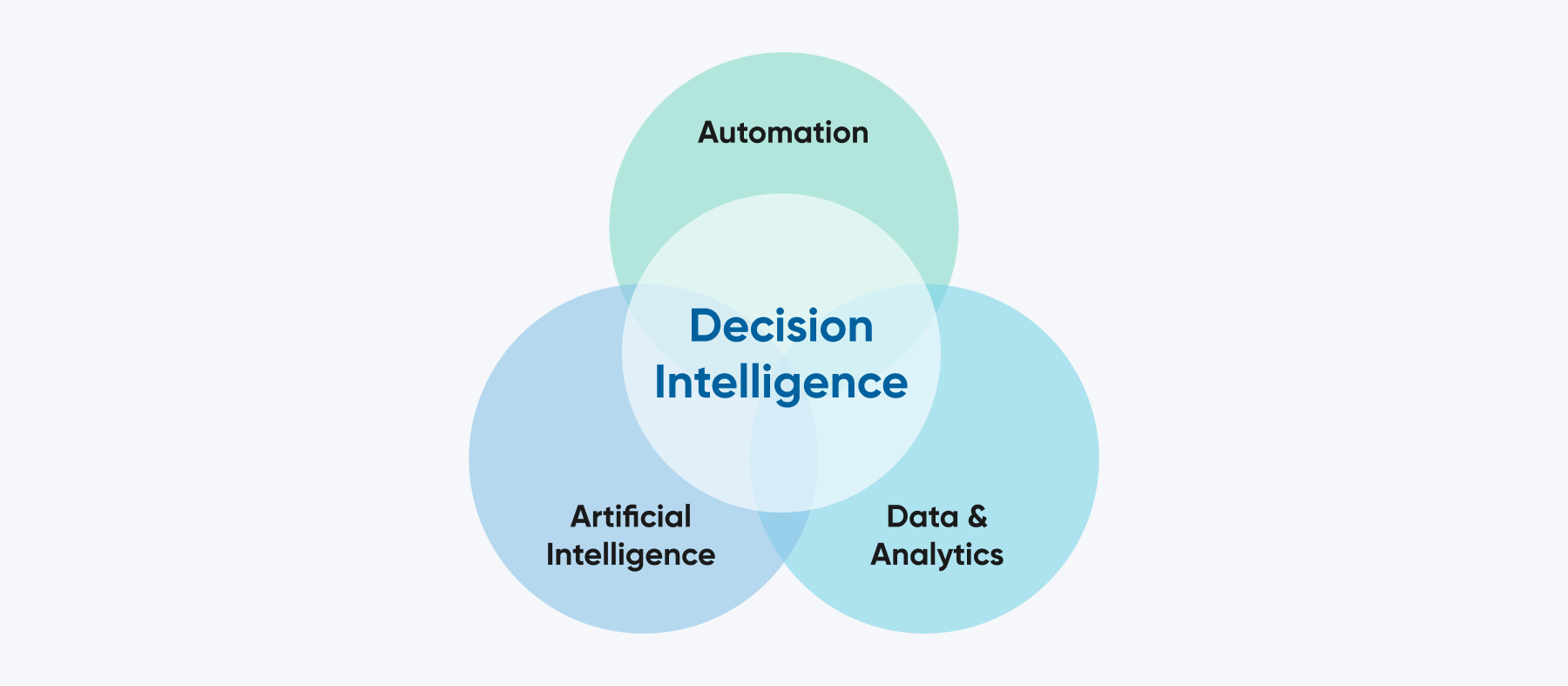
Traditional decision-making, reliant on human interpretation of data, can’t keep pace with today’s speed and complexity. What businesses need now is decision intelligence — an intelligent, scalable solution to continuously optimize decisions. DI not only recommends the best course of action but learns from outcomes and executes decisions in real time. The result: faster, more consistent, and more governed decision-making across the enterprise.
Key aspects of decision intelligence:
- Data-driven decisions: Uses real-time, historical, structured, and unstructured data to inform actions.
- AI and automation: Applies logic and rules to automate decisions, reducing manual work and improving speed.
- Context-aware intelligence: Considers business goals, constraints, and environments in each recommendation.
- Scenario planning: Enables simulation of multiple outcomes to support smarter choices.
- Continuous learning: Improves decision quality through feedback loops.
Decision intelligence repositions humans as decision architects who design how decisions are made. It operates at three levels of AI involvement, depending on the type of data:
- Decision support (human in the loop): AI provides insights and performs simulations with alternative paths (e.g., scenario modeling for pricing decisions)
- Decision augmentation (human on the loop): AI provides recommendations with full context and possible impact, and a human makes the final call to execute, change or reject the decision (e.g., recommendations for supplier sourcing)
- Decision automation (human out of the loop): AI autonomously makes decisions and takes action within predefined constraints, with full auditability of actions (e.g., fully executed logistics optimization).
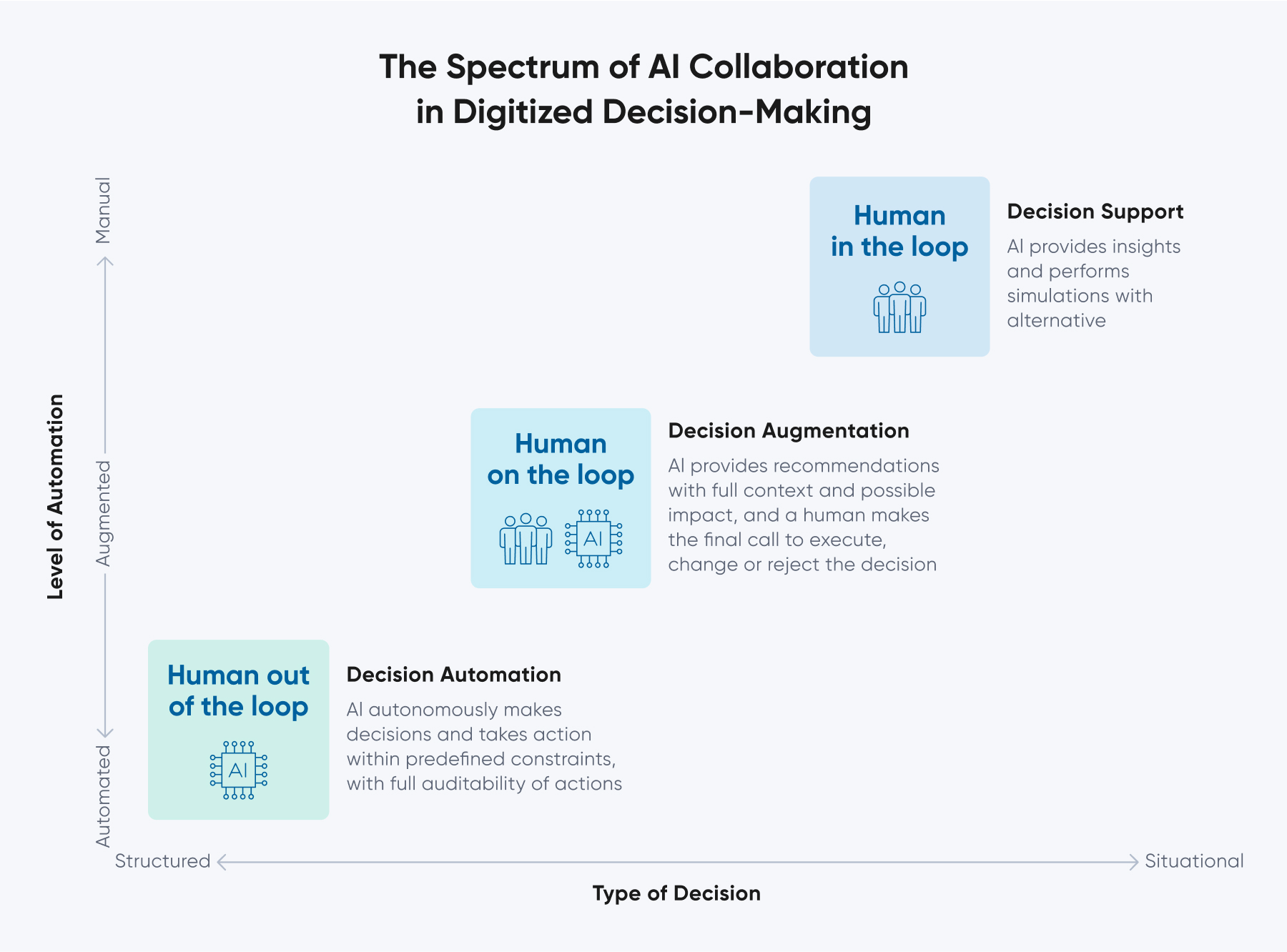
At every level, transparency and feedback improve decision quality. DI turns decision-making into a structured, scalable process that drives measurable business results.
Benefits, Capabilities, Use Cases
Why is decision intelligence important? What are its benefits?
Decision intelligence is essential for businesses facing a surge in decision volume and complexity in today’s fast-moving environment. It enables smarter, synchronized decision-making at scale by linking data, recommendations, and actions — optimizing decisions across the value chain and helping organizations stay agile and competitive.
Key benefits include:
- Improved decision accuracy and granularity. DI analyzes all data — structured and unstructured — and applies analytics and AI to improve decision quality. It supports, augments, or automates decision-making at a level of detail and accuracy human judgment alone can’t achieve.
- Increased decision speed and efficiency. DI reduces manual effort by providing real-time recommendations and automating routine decisions, scaling decision velocity across the organization.
- Reduced risk and uncertainty. DI simulates scenarios, surfaces potential risks, and helps implement proactive strategies — leading to better compliance, fewer surprises, and smarter investments.
- Consistency and enhanced collaboration. DI provides a single version of the truth, accessible to all teams via dashboards and natural language interfaces, ensuring aligned and consistent decision logic.
- Connected decisions and continuous learning. DI links decisions across functions and continuously learns, optimizing decision-making at scale.
Decision intelligence is AI designed to drive tangible business value and measurable ROI. In supply chains, for instance, it cuts costs, reduces waste, boosts efficiency, improves inventory control, and increases service levels — all through more intelligent, automated decision-making:
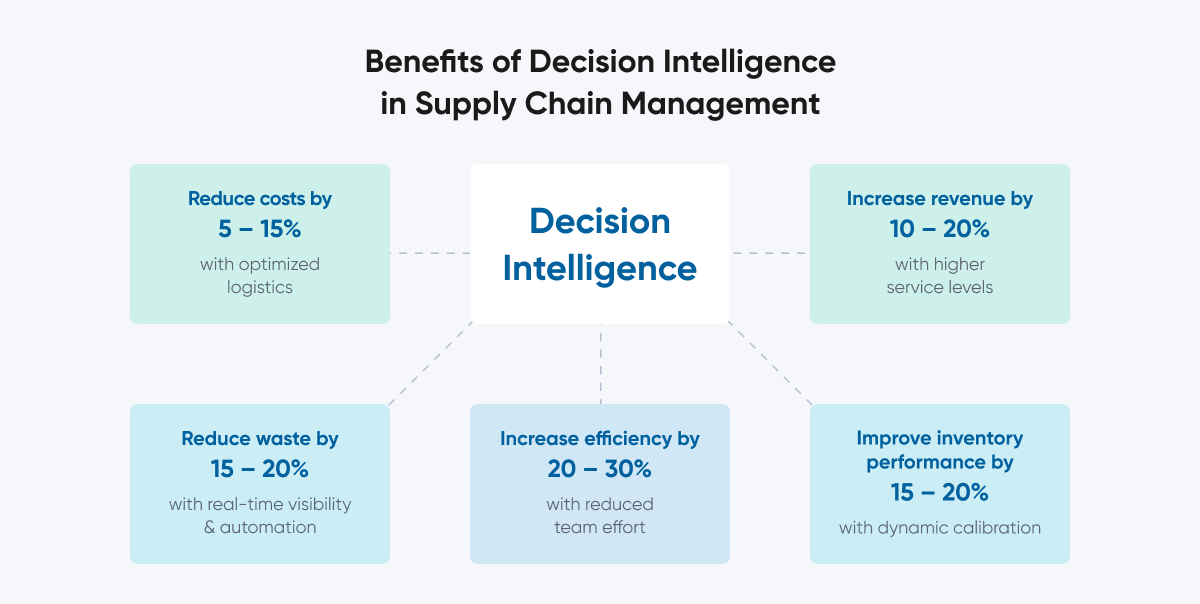
In short, DI enables faster, better decisions by fusing AI’s scale with human judgment — helping organizations shift from reactive choices to proactive strategy execution.
Learn more about how Aera customers are using decision intelligence.
What is a decision intelligence platform?
Gartner defines decision intelligence platforms (DIPs) as "software used to create solutions that support, automate and augment decision making of humans or machines, powered by the composition of data, analytics, knowledge, and artificial intelligence (AI) techniques." These platforms bridge data and execution, transforming insights into timely action.
At a minimum, a true decision intelligence platform should incorporate all of these crucial components:
- Data. It aggregates and harmonizes data from multiple sources — structured databases, unstructured text, IoT devices, cloud apps — to provide a comprehensive view. It extracts and unifies billions of transactions from enterprise systems and external sources into an open, composable, transactional decision data model.
- Automation. It mirrors the dynamic thinking behind human decisions by applying transparent business rules and AI-driven logic. The platform can automate execution where appropriate, while still allowing humans to review and control as needed.
- Composability. It’s modular, flexible, and configurable — enabling businesses to adapt quickly without overhauling systems. It allows organizations to assemble decision flows, integrate AI models, and build custom interfaces for specific needs.
In addition, an advanced decision intelligence platform incorporates these components:
- Intelligence. It leverages AI to forecast outcomes using historical and real-time data. It supports decision automation, augmentation, and support by continuously sensing conditions, analyzing shifts, and learning from outcomes. Over time, this creates a self-improving, real-time learning loop that evolves decision models with changing business needs.
- Engagement. Leveraging agentic AI, it engages users through natural language and interactive UX. It explains its reasoning, provides full data traceability, and fosters trust by showing the “why” behind every recommendation — down to the transaction level.
In addition to these attributes, many decision intelligence platforms provide intuitive dashboards, visual analytics, and interactive reports that make data insights accessible to business users without requiring deep technical expertise.
Read more in the Gartner Market Guide for Decision Intelligence Platforms.
What are the key capabilities of a decision intelligence platform?
A robust DI platform combines capabilities across data, AI, workflow automation, and user interaction:
- Data Integration & Real-Time Processing
- Structured and unstructured data ingestion
- Data integration and streaming from multiple sources
- Data harmonization
- Decision Composibility
- Decision modeling and orchestration design
- Advanced analytics and composite AI/ML capabilities
- Simulation and modeling capabilities to test potential decisions
- AI agents for decision reasoning
- Recommendation engines to suggest optimal choices
- Decision-Centric Engagement
- Decision-centric interfaces
- Natural language processing for conversational insights
- Simulation and modeling capabilities to test potential decisions
- Visualization techniques to provide contextual information
- Learning & Auditability
- Continuous learning from every decision made
- Transparency and auditing of decision types, number of decisions, outcomes, and approvals
- Enterprise-Scale Deployment & Security
- Assurance of data governance, regulatory compliance, and role-based access control
- Scaling to handle complex, multi-layered decision processes across global operations
Together, these capabilities help companies standardize and scale decision-making across functions and time horizons. DI platforms create consistent, repeatable processes that allow organizations to adapt quickly, simulate outcomes effectively, and track performance with clarity. By relying on data and logic rather than intuition, they reduce bias and improve objectivity. This results in a more agile, transparent, and measurable system of intelligence — one that aligns strategy and action to accelerate enterprise-wide transformation.
Who uses decision intelligence, and what problems does it solve across industries?
Decision intelligence platforms are used across a wide variety of sectors — especially those that rely on high volumes of data, rapid decision cycles, and complex value chains.
All Manufacturing
- Demand Forecasting: Predicting demand fluctuations and adjusting inventory levels accordingly.
- Inventory Waste Management: Reducing waste and markdowns by identifying slow-moving or expiring products and recommending mitigations.
- Logistics Optimization: Determining the most efficient transportation routes to reduce costs, delivery times and carbon emissions.
- Warehouse Management: Automating restocking and optimizing warehouse space utilization.
- Control Tower Visibility: Providing real-time insights to manage disruptions proactively.
Retail & Consumer Goods
- Price Optimization: Dynamically adjusting pricing based on demand, competition, and inventory levels.
- Inventory Replenishment: Ensuring products are available when and where they are needed.
- Claims Management: Extracting unstructured claims data, validating claims, and automating claims processing.
LifeSciences & Pharmaceuticals
- Drug Supply Chain Management: Ensuring the timely distribution of medications.
- Clinical Trial Optimization: Identifying the best patient cohorts and trial locations.
Energy & Utilities
- Sustainability Planning: Helping businesses meet carbon reduction goals efficiently.
Financial Services & Banking
- Fraud Detection: Identifying fraudulent transactions in real time.
- Risk Assessment: Automating credit and loan approval processes.
In particular, large companies with massively complex operations provide excellent use cases for decision intelligence — both to harmonize all the data they generate across multiple systems, and to digitize, augment, and automate decision-making at scale. By addressing inefficiencies, improving forecasting, and mitigating risks, decision intelligence platforms enable organizations across these industries to enhance performance, reduce costs, and drive better strategic outcomes.
Learn more about how Aera customers are using decision intelligence.
Differences from Other Tools & Disciplines
How is decision intelligence different from other analytical tools?
Decision intelligence goes beyond traditional analytics. While business intelligence (BI) tools focus on describing what happened — through reports, dashboards, and historical trends — DI integrates predictive and prescriptive analytics to guide what should happen next.
And unlike static BI dashboards that require human interpretation, DI platforms use AI to adapt in real time. They simulate scenarios, optimize recommendations, and align decisions with strategic goals — making them more dynamic and actionable. Finally, DI transforms data into intelligent decision-making that learns and improves continuously.
How decision intelligence (DI) differs from business intelligence (BI):
Business Intelligence (BI) | Decision Intelligence (DI) | |
---|---|---|
Focus | Provides reporting and analytics | Provides decision support, augmentation and automation |
Output | Presents data visualizations | Gives predictions, recommendations, and automated actions |
Scope | Is descriptive and diagnostic | Is prescriptive and proactive |
Learning | Generates static reports without learning | Learns continuously from decision outcomes |
In brief: DI platforms don’t just analyze data — they act on it. They automate routine decisions and support strategic ones, all in real time.
How are planning solutions different from decision intelligence platforms?
Planning tools and DI platforms both support decisions — but differ in adaptability, intelligence, and execution.
How DI platforms differ from planning solutions:
Planning Solutions | DI Platforms | |
---|---|---|
Primary goal | Build static plans using historical data, assumptions, and forecasting models | Enable real-time decision-making, automation, and continuous learning from outcomes |
Scope | Support structured, periodic planning cycles and scenario analysis | Cover end-to-end execution of decisions – from strategic to operational and situational. |
Time Horizon | Focused on long-term and periodic planning cycles. | Designed for real-time, near-term, and long-range decisions. |
Execution of Plans | Generate plans that require manual implementation and tracking. | Recommend optimal decisions and take autonomous action when needed. |
Adaptability | Plans often become outdated quickly as conditions change. | Dynamically adjust to real-time data, events, and signals. |
Data Processing | Use historical and structured data for forecasting | Process real-time, structured, and unstructured data from diverse sources |
AI & Machine Learning | Often limited to forecasting and optimization models | Use AI for continuous learning, automation, and contextual decision-making |
Decision Learning | Do not learn from past plans or decisions | Continuously learn from decisions and outcomes, improving over time |
Tech Stack Integration | Typically require manual integration with execution systems. | Seamlessly connect with ERP, CRM, SCM, and external platforms. |
Human & AI Collaboration | Mostly human-driven, requiring analysts to interpret and act on plans | Enable human–AI collaboration, with AI augmenting or automating decisions |
In brief: Planning tools simply forecast. DI platforms recommend and act — in real time, across the enterprise, with intelligence that evolves.
What is the difference between decision intelligence and data science?
Decision intelligence and data science work together but serve different goals. Data science focuses on extracting insights from data using statistics, machine learning, and AI. It identifies patterns, predicts trends, and builds models to explain complex datasets. DI takes those insights and turns them into action — integrating them into decision-making to deliver optimized outcomes.
Key distinctions include:
- Objective. Data science generates insights; DI uses them to improve decisions.
- Scope. Data science centers on analysis and modeling; DI embeds insights into workflows, automation, and strategy.
- End-User Impact. Data science supports analysts; DI empowers decision-makers and systems across the business.
- Automation. DI emphasizes real-time, AI-driven action; data science often requires human interpretation.
Data science builds understanding. Decision intelligence ensures that understanding drives real-world decisions that matter.
The Role of Artificial Intelligence in Decision Intelligence
How is artificial intelligence related to decision intelligence?
Decision intelligence applies artificial intelligence to optimize decision-making. AI is the broader field — machines that learn, reason, and predict — while DI is a targeted application of those capabilities.
Here’s how AI powers DI:
- Predictive Capabilities. AI analyzes past and present data to forecast outcomes and recommend next steps.
- Decision Automation. AI handles routine and complex choices, cutting manual work and boosting speed.
- Continuous Learning. Machine learning models improve over time through feedback and outcomes.
- Cognitive Augmentation. AI delivers insights, flags anomalies, and explains itself — fostering trust and transparency.
- Real-Time Data Processing. From structured to unstructured inputs, AI enables instant analysis and response.
AI includes machine learning, optimization, rules engines, NLP, and graph technologies. DI blends these into composite AI — a powerful stack that enhances accuracy, adaptability, and impact. AI is the engine; decision intelligence makes it drive with purpose, aligning actions with strategy and human judgment.
What is agentic AI, and how does it enhance decision intelligence beyond other AI methods?
Agentic AI is the next leap forward. It doesn’t replace predictive or generative AI — it amplifies them. It creates a more cohesive, capable decision-making system.
- Predictive AI offers foresight with forecasts and scenario models.
- Generative AI enables intuitive interaction and produces clear, user-friendly content.
- Agentic AI fuses these strengths with autonomous reasoning and execution.
Designed to make complex decisions and adapt on its own, agentic AI becomes an engine of self-optimization. It doesn’t just recommend — it acts. It’s ideal for domains like supply chains, pricing, and operations, where fast, fine-tuned decisions are critical. With agentic AI, DI platforms evolve from reactive systems into proactive, self-improving ecosystems that drive innovation and efficiency.
Take this example: predictive AI spots a demand surge; generative AI helps users interpret the data; agentic AI assesses the impact, reallocates inventory, and initiates action — keeping humans informed along the way. When combined with decision intelligence, agentic AI empowers enterprises to build intelligent, adaptive systems that move with the market, not behind it. Agility becomes built-in.
Deploying Decision Intelligence with a Platform
How can companies get started with decision intelligence?
To deploy decision intelligence effectively, companies should take a structured approach:
- Identify Decision-Making Challenges. Pinpoint where decisions are slow, error-prone, or overly reliant on gut feel.
- Assess Data Availability & Quality. Ensure access to clean, relevant, and comprehensive data.
- Implement a Decision Intelligence Platform. Choose a platform that integrates with existing systems and supports analytics, automation, and AI-based decision support.
- Train Teams & Build a Data-Driven Culture. Upskill employees and promote a mindset that values data over intuition.
- Start with High-Impact Use Cases. Focus on areas like forecasting, risk, or supply chain to demonstrate early value.
- Monitor, Measure & Refine. Track outcomes, fine-tune models, and continuously improve.
This approach helps organizations shift to faster, smarter, and more efficient decision-making.
Here are real-world examples of companies starting with a clear business challenge, applying DI, and achieving stronger outcomes:
- A global steel manufacturer uses DI to identify root causes of late shipments, recommend actions, and improve communications and customer service when delays occur.
- A petrochemical company deploys DI for better shipping performance, gaining real-time visibility across its supply chain, including third-party operations.
- A global pharmaceutical company uses DI to generate ATP (available-to-promise) estimates across diverse products by syncing data from multiple ERP systems — helping customers know when an order has been received, when it will be shipped, and when to expect it to be delivered.
Learn more about how Aera customers are using decision intelligence.
What is a decision intelligence skill?
A decision intelligence skill is a modular capability built into a decision intelligence platform. It automates or assists decision-making in a specific area, functioning like AI skills in virtual assistants — offering contextual recommendations with transparency and ongoing learning.
Each skill delivers a targeted solution with four core capabilities:
- Data Integration. Pulls and harmonizes data into a common model.
- Analytics & Modeling. Applies ML, simulations, and other calculations tailored to the decision at hand.
- Digitized Logic & Flow. Encodes business rules and user interactions.
- Execution. Pushes decisions back into operational systems.
Examples include:
- Logistics. Optimizes routes and modes for cost, speed, service, and sustainability.
- Demand Forecasting. Predicts trends using historical patterns and external variables.
- Inventory Balancing. Minimizes stockouts and aging inventory, adjusting to real-time supply-demand shifts.
- Availability to Promise. Analyzes inventory and lead times to offer accurate delivery commitments.
- Tariff Impact Mitigation. Models trade scenarios and finds lower-cost sourcing alternatives.
Skills enhance DI platforms by allowing businesses to customize decision-making capabilities according to their needs. As you add more skills, you can reuse common core elements and exchange information, making skills smarter and more efficient over time.
What is self-service decision intelligence?
Self-service decision intelligence puts DI tools in the hands of business users — no deep technical expertise required. With intuitive interfaces and low-code or no-code setup, non-technical teams can act on insights faster.
Self-service DI enables users to:
- Build and test decision models without coding.
- Understand AI-driven recommendations via clear dashboards.
- Run scenarios and compare decision paths.
- Automate decisions with minimal IT involvement.
This accessibility broadens adoption and drives a culture of data-backed decision-making, enhancing agility across the organization.
Where does decision intelligence fit in the enterprise stack?
Decision intelligence forms a crucial layer in the enterprise technology stack. It connects raw data, AI insights, and execution — bridging analytics, automation, and operational systems.
As organizations embrace DI, the stack will evolve into something simpler and more streamlined:
- Systems of Record (ERP, CRM, POS, etc.) become more automated, reducing manual work.
- A System of Intelligence emerges to coordinate decisions across functions, blending AI insights with human input.
With this shift, decision-making will become fluid and dynamic, allowing humans to operate in, on, or out of the loop, depending on the level of automation required.
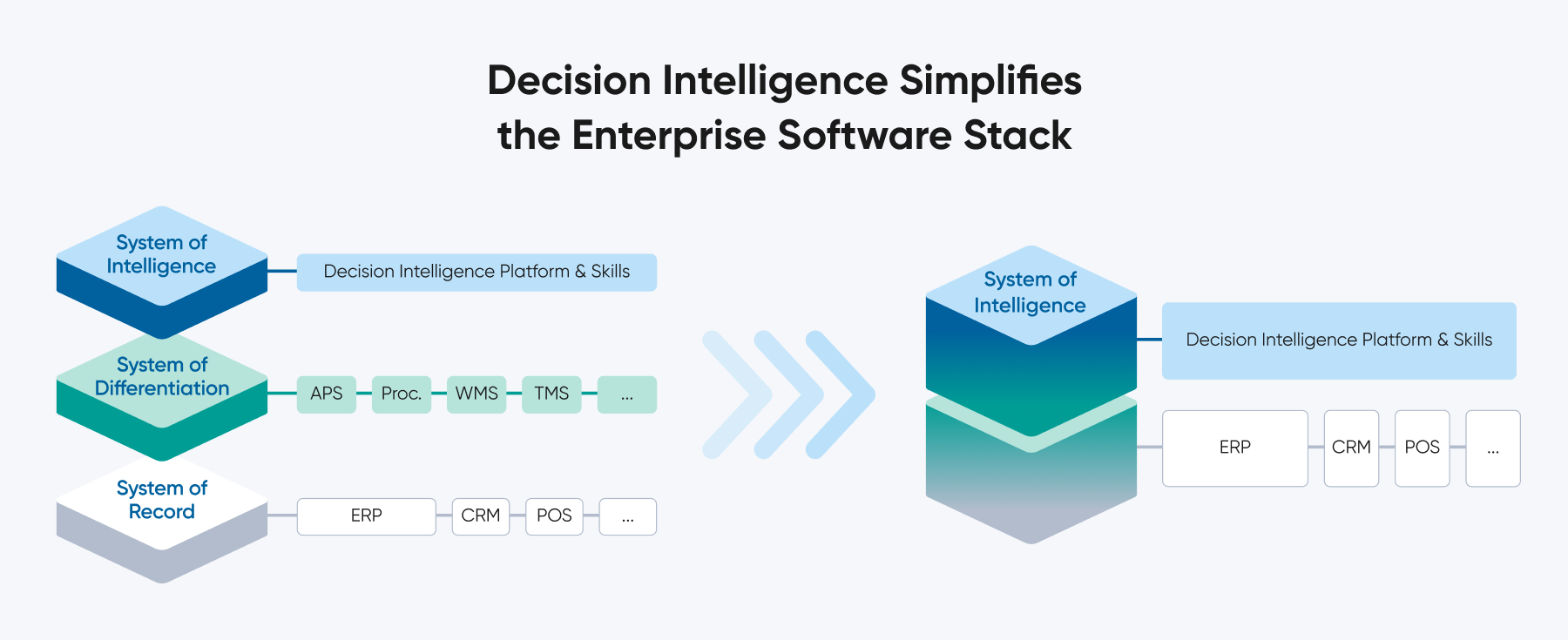
This integration shifts decision-making from rigid and reactive to fluid and dynamic. Humans can stay in the loop, step out, or oversee as needed.
By integrating across the stack, decision intelligence enables faster, more accurate, and automated decisions — empowering organizations to boost performance, reduce risk, and stay competitive in a fast-moving world.
Ready to Make Smarter Decisions?
The way businesses make decisions is evolving. Will your company lead the change or lag behind? Decision intelligence offers a clear path to more strategic, data-driven, and automated decision-making. Explore how decision intelligence can transform your business.